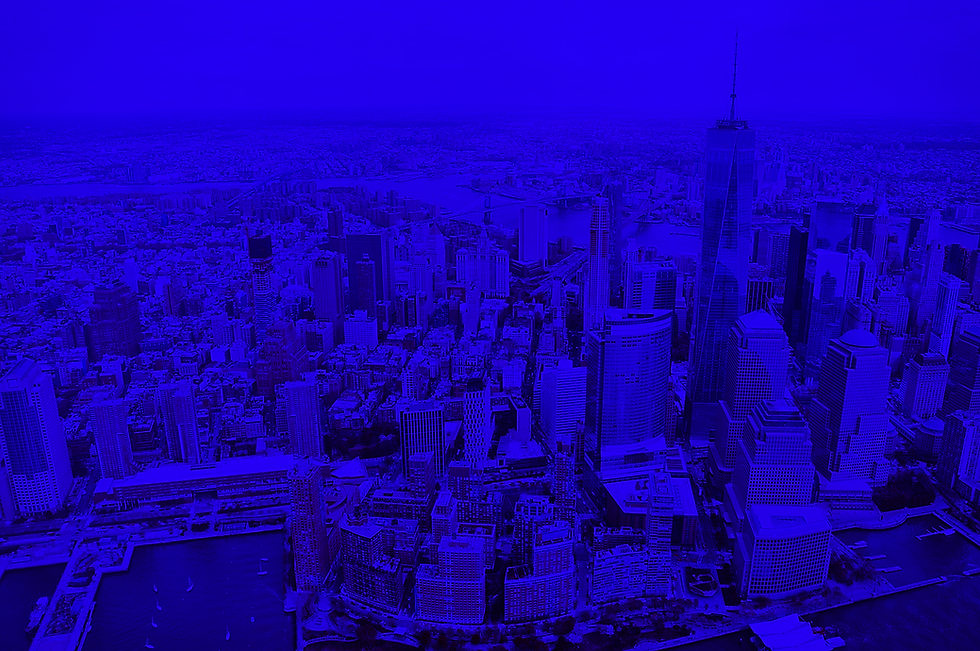
WCCI 2022 SPECIAL SESSION ON
Data-Driven Decision Support for Streaming Data with Uncertainties
July 18-23, Centro Congressi, Padova, Italy
Background
Machine Learning for streaming data has enjoyed tremendous growth and exhibited a wealth of developments, at both the conceptual and application level, in recent years. It comes with a number of algorithms with successful experiments reported thoroughly in the literature. In particular, there have been numerous successful realizations of stream-based machine learning methods in application areas, including e-service, finance, transportation, weather forecasting, telecommunications, product pricing, and so on. Handling streaming data in real-time is highly visible in both machine learning and data science fields.
Existing data-driven decision support systems focus on stationary data. Few decision support systems have been reported in existing studies addressing uncertainties in streaming data such as the distributional changes, evolving features, new labels, temporal dependency, etc. Namely, it still lacks autonomous and self-adaptive decision support systems in the literature that can provide real-time accurate decision solutions for high-volume streaming data.
Aims
This special session is to provide a forum for researchers in the decision making field and the stream learning field to share the latest advantages in theories, algorithms, and applications on streaming data-driven decision support systems. Specifically, this session will focus on real-time decision support for streaming data that may contain uncertainties such as concept drift, evolving features or labels, noisy time series, imbalanced labels, temporal dependency, etc. These research directions include several hot topics in the field of decision making and stream learning such as real-time decision support, adaptive decision support systems, decision-making under uncertainty, concept drift, nonstationary environment and multiple stream learning.
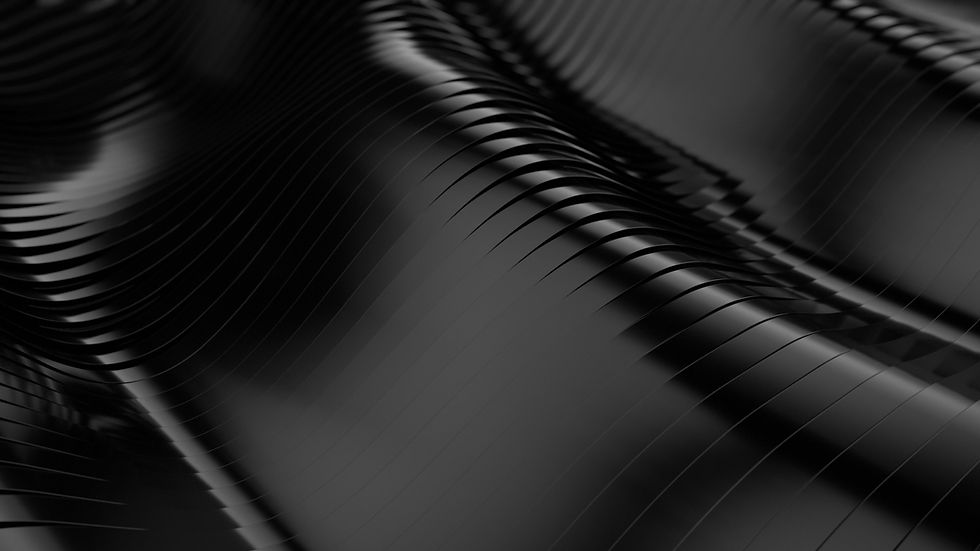
Detailed Descriptions
We welcome submissions in but not limted to the following aspects
Adaptive decision support under concept drift.
In this case, the decision support methodologies should be able to overcome the decision accuracy degrade due to the data distribution changing problem in a data stream. Related research topics include but are not limited to
-
Concept drift detection, understanding and adaptation
-
Incremental learning
-
Online learning
-
Lifelong learning
-
Continue learning
Autonomous decision support under evolving data
In this case, the decision support methodologies should be able to handle evolved features or labels, such as newly appeared features or labels in a data stream. Related research topics include but are not limited to
-
Reinforcement learning on streaming data
-
Active learning
-
Evolutionary learning
Robust decision support under noisy or imbalanced data
In this case, the decision support methodologies should be able to handle noisy data or imbalanced data. Related research topics include but are not limited to
-
Imbalance learning
-
Online denoising
Real-world applications of decision making for streaming data
Related research topics include but are not limited to
-
Real-time streaming data prediction
-
Real-time decision making
-
Real-time recommender systems
Decision support for streaming data with other uncertainties
Related research topics include but are not limited to
-
Real-time decision support for streaming data with temporal dependency
-
Real-time decision support for streaming data with missing data
-
Real-time decision support for streaming data with abnormal data
Decision support for multiple data streams
In this case, the decision support methodologies should be able to handle multiple related data streams in real-time as a whole. Related research topics include but are not limited to
-
Concept drift detection, understanding, adaptation for multiple data streams
-
Learning dynamic structures for multiple streams
-
Online transfer learning between multiple streams
Submission Details
Submission System
WCCI 2022 adopts Microsoft CMT as the submission system, available at the following link:
Speakers
Important Dates
It is our great pleasure to invite you to the bi-annual IEEE World Congress on Computational Intelligence (IEEE WCCI), which is the largest technical event in the field of computational intelligence. The IEEE WCCI 2022 will host three conferences: The 2022 International Joint Conference on Neural Networks (IJCNN 2022 – co-sponsored by International Neural Network Society – INNS), the 2022 IEEE International Conference on Fuzzy Systems (FUZZ-IEEE 2022), and the 2022 IEEE Congress on Evolutionary Computation (IEEE CEC 2022) under one roof. It encourages cross-fertilization of ideas among the three big areas and provides a forum for intellectuals from all over the world to discuss and present their research findings on computational intelligence.
IEEE WCCI 2022 will be held in Padua, Italy, one of the most charming and dynamic towns in Italy. It is located in the Veneto region, in Northern Italy. The historical center boasts a wealth of medieval, renaissance and modern architecture. Here a fascinating mix of historic and new, of centuries-old traditions and metropolitan rhythms creates a unique atmosphere. The medieval palaces, churches and cobbled streets emanate a sense of history. Padua features rich historical and cultural attractions, such as “Prato della Valle”, the largest square in Europe; the famous Scrovegni Chapel painted by Giotto; the Botanical Garden that is a UNESCO Word Heritage; the University of Padua, that is the second oldest university in Italy (1222) celebrating, in 2022, 800 years of history.
See the important dates below:
-
Paper submission:
-
Title and Abstract: January 31, 2022 (11:59 PM AoE) New submissions cannot be created past this deadline.
-
Complete Paper (pdf): February 31, 2022 (11:59 PM AoE) STRICT DEADLINE
-
-
Notification of acceptance: April 26, 2022
-
Final paper submission: May 23, 2022
-
Conference: 18 - 23 July, 2022
Organisers
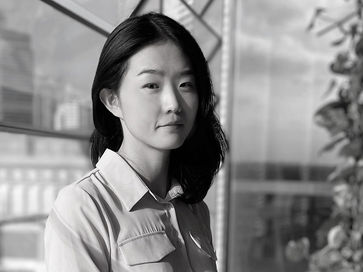
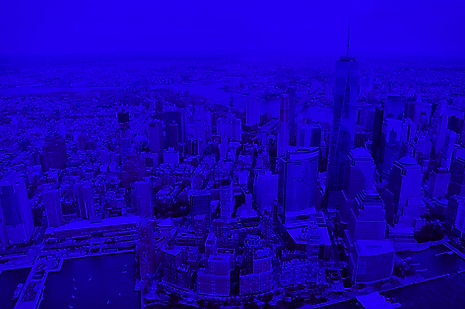
If you have questions about the submission/registration process, don’t hesitate to reach out.
Yiliao Song (Yiliao.Song@uts.edu.au)